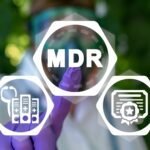
How AI and Machine Learning Are Enhancing the Accuracy of Diagnostic Imaging Devices
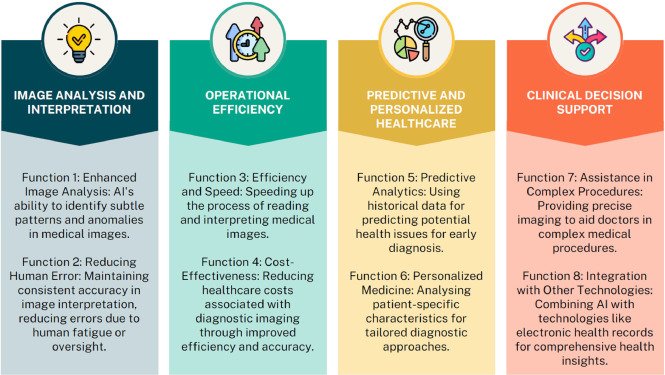
How AI and Machine Learning Are Enhancing the Accuracy of Diagnostic Imaging Devices:
Overview: Artificial Intelligence (AI) and Machine Learning (ML) are rapidly transforming the field of diagnostic imaging, significantly improving the accuracy, efficiency, and overall effectiveness of imaging devices. AI and ML technologies enable imaging systems to analyze and interpret medical images with greater precision, detect subtle patterns, and assist healthcare professionals in making faster, more reliable diagnoses.
AI and ML have become crucial tools in enhancing the capabilities of diagnostic imaging devices across various modalities, such as X-rays, CT scans, MRIs, ultrasounds, and mammography. These advancements not only help reduce diagnostic errors but also contribute to better patient outcomes, especially in settings where radiologist expertise may be scarce.
Key Ways AI and Machine Learning Enhance Diagnostic Imaging:
- Improved Image Interpretation:
- AI algorithms, particularly deep learning models, are designed to recognize patterns and features in medical images that might be difficult for the human eye to detect. ML models can analyze images at the pixel level and identify subtle anomalies that may go unnoticed by radiologists.
- Example: AI algorithms used in mammography have been shown to detect breast cancer more accurately by identifying patterns associated with malignancy, even in dense breast tissue that can be challenging for traditional imaging to assess.
- AI algorithms, particularly deep learning models, are designed to recognize patterns and features in medical images that might be difficult for the human eye to detect. ML models can analyze images at the pixel level and identify subtle anomalies that may go unnoticed by radiologists.
- Enhanced Detection of Abnormalities:
- Machine learning systems are increasingly used to detect early-stage diseases and abnormalities, including tumors, lesions, and other pathological conditions, at much earlier stages than traditional diagnostic methods.
- Example: AI-powered tools used with CT scans can detect lung cancer at its earliest stages by recognizing minute signs such as nodules or calcifications. These early detections allow for earlier interventions and better survival rates.
- Machine learning systems are increasingly used to detect early-stage diseases and abnormalities, including tumors, lesions, and other pathological conditions, at much earlier stages than traditional diagnostic methods.
- Automated Image Segmentation:
- Segmentation is the process of identifying and isolating specific regions of interest (such as organs, tumors, or lesions) within an image. AI and ML techniques, especially deep learning models, can automatically segment and classify various structures in medical images with high accuracy, reducing the time and effort required by radiologists.
- Example: In MRI scans of the brain, AI algorithms can automatically delineate areas affected by multiple sclerosis, stroke, or neurodegenerative diseases, making it easier for doctors to assess the extent of damage or progression.
- Segmentation is the process of identifying and isolating specific regions of interest (such as organs, tumors, or lesions) within an image. AI and ML techniques, especially deep learning models, can automatically segment and classify various structures in medical images with high accuracy, reducing the time and effort required by radiologists.
- Quantitative Analysis and Measurement:
- AI systems can be programmed to perform quantitative analyses of medical images, such as measuring tumor size, tissue density, or blood vessel structures, and provide precise measurements that are crucial for diagnosis and treatment planning.
- Example: In cardiac imaging, AI-powered systems can quantify heart wall motion, chamber volumes, and ejection fractions from echocardiograms or CT angiograms, helping cardiologists assess heart function with greater accuracy and speed.
- AI systems can be programmed to perform quantitative analyses of medical images, such as measuring tumor size, tissue density, or blood vessel structures, and provide precise measurements that are crucial for diagnosis and treatment planning.
- Reduced Diagnostic Time and Increased Efficiency:
- AI can streamline the process of reviewing and interpreting images by automatically flagging abnormal findings, prioritizing cases that require immediate attention, and providing radiologists with a preliminary analysis. This reduces the time spent reviewing images and allows healthcare professionals to focus on the most critical cases, improving overall efficiency.
- Example: AI-driven triage systems are being integrated with X-ray and CT imaging devices to quickly assess and prioritize images based on the severity of findings. This is particularly useful in emergency situations, such as in trauma care or stroke assessment.
- AI can streamline the process of reviewing and interpreting images by automatically flagging abnormal findings, prioritizing cases that require immediate attention, and providing radiologists with a preliminary analysis. This reduces the time spent reviewing images and allows healthcare professionals to focus on the most critical cases, improving overall efficiency.
- Decision Support for Clinicians:
- AI algorithms serve as decision-support tools, assisting radiologists and clinicians by providing additional insights and recommendations based on the analysis of images. AI doesn’t replace the radiologist, but it enhances their decision-making capabilities, leading to more accurate and timely diagnosis.
- Example: AI models can analyze chest X-rays or CT scans for signs of COVID-19 pneumonia, guiding healthcare workers to make faster and more informed decisions regarding treatment and isolation protocols.
- AI algorithms serve as decision-support tools, assisting radiologists and clinicians by providing additional insights and recommendations based on the analysis of images. AI doesn’t replace the radiologist, but it enhances their decision-making capabilities, leading to more accurate and timely diagnosis.
- Integration with Other Patient Data:
- AI and ML can also integrate diagnostic imaging data with electronic health records (EHR), genetic information, and other clinical data to create a comprehensive view of a patient’s condition. This integration enhances the predictive capabilities of diagnostic devices, allowing for personalized care and more accurate prognostications.
- Example: AI-powered platforms are combining imaging data with genome sequencing to create personalized cancer treatment plans. For instance, combining CT images of tumors with genetic data helps doctors identify the most effective treatment regimens for specific types of cancer.
- AI and ML can also integrate diagnostic imaging data with electronic health records (EHR), genetic information, and other clinical data to create a comprehensive view of a patient’s condition. This integration enhances the predictive capabilities of diagnostic devices, allowing for personalized care and more accurate prognostications.
- Improving Radiologist Workflow and Reducing Fatigue:
- Diagnostic imaging devices enhanced by AI also help alleviate radiologist burnout by reducing the cognitive load required for repetitive and time-consuming tasks like image review and interpretation. AI can handle routine cases, allowing radiologists to focus on more complex diagnoses and reducing the overall fatigue associated with high-volume image analysis.
- Example: AI-assisted systems can automate routine reads of chest X-rays or spine imaging, leaving radiologists to focus on more complicated and ambiguous cases.
- Diagnostic imaging devices enhanced by AI also help alleviate radiologist burnout by reducing the cognitive load required for repetitive and time-consuming tasks like image review and interpretation. AI can handle routine cases, allowing radiologists to focus on more complex diagnoses and reducing the overall fatigue associated with high-volume image analysis.
- AI-Powered Virtual Radiology Assistants:
- Several AI solutions are designed to function as virtual assistants, which can help guide radiologists through the diagnostic process. These AI assistants can suggest potential diagnoses, provide confidence scores for findings, and assist with report generation, improving the consistency and accuracy of radiology reports.
- Example: AI tools like Zebra Medical Vision offer assistive technologies that can support radiologists by flagging potential pathologies in images and recommending further steps based on previous cases and medical literature.
- Several AI solutions are designed to function as virtual assistants, which can help guide radiologists through the diagnostic process. These AI assistants can suggest potential diagnoses, provide confidence scores for findings, and assist with report generation, improving the consistency and accuracy of radiology reports.
Applications of AI in Various Imaging Modalities:
- X-Ray:
- AI is being used to enhance X-ray imaging by automatically detecting conditions like pneumonia, fractures, cardiomegaly (enlarged heart), and osteoporosis. AI systems can quickly identify these issues and help prioritize cases based on severity, which is especially beneficial in emergency settings or high-volume clinics.
- MRI (Magnetic Resonance Imaging):
- AI in MRI is used to speed up image acquisition, reduce noise, and improve the resolution of scans. For example, AI can help in the early diagnosis of brain tumors, multiple sclerosis, Alzheimer’s disease, and musculoskeletal disorders by analyzing the fine details in MRI scans that could be overlooked.
- CT Scans (Computed Tomography):
- AI and ML are increasingly integrated into CT scans to enhance image quality and enable early detection of diseases such as cancer, stroke, and heart disease. AI algorithms can identify early signs of lung cancer or pulmonary embolism and automatically highlight abnormalities in the images for radiologists to review.
- Ultrasound:
- In ultrasound imaging, AI tools can assist in real-time analysis of images, such as identifying abnormalities in fetal development, diagnosing heart conditions, or monitoring organ health. AI also helps in standardizing ultrasound scans, making it easier to identify important features even in less experienced hands.
- Example: AI can assist in identifying fetal abnormalities in pregnancy scans, guiding clinicians in making faster, more accurate decisions during prenatal care.
- In ultrasound imaging, AI tools can assist in real-time analysis of images, such as identifying abnormalities in fetal development, diagnosing heart conditions, or monitoring organ health. AI also helps in standardizing ultrasound scans, making it easier to identify important features even in less experienced hands.
- Mammography:
- AI plays a significant role in breast cancer detection, with algorithms that can analyze mammograms for abnormalities, such as masses, calcifications, and asymmetries. AI systems can reduce false positives and false negatives, improving early detection and ensuring better outcomes for women at risk of breast cancer.
Challenges and Limitations:
- Data Quality and Diversity:
- AI systems rely on large, high-quality datasets for training. However, the quality and diversity of training data can impact the accuracy and generalizability of AI models. A lack of diverse datasets, especially from underrepresented groups, can lead to biases and misdiagnoses in specific populations.
- Regulatory Approval and Standards:
- AI models for diagnostic imaging must undergo rigorous validation and regulatory approval, such as from the FDA (in the US) or CE marking (in Europe). Ensuring that AI technologies meet regulatory standards and are clinically validated for real-world use is essential for their widespread adoption.
- Integration into Existing Systems:
- Integrating AI-powered diagnostic imaging devices into existing hospital infrastructure can be challenging. AI tools need to be compatible with current PACS (Picture Archiving and Communication Systems) and EHR systems to ensure smooth workflows and avoid disruptions.
- Ethical Considerations:
- The use of AI in healthcare raises ethical concerns, such as data privacy, transparency, and accountability. It’s essential to ensure that AI models are interpretable, and that decisions made by AI systems are explainable to both patients and clinicians.